Examples
abound of how large corporations have harnessed the power of big data analytics
across various aspects of their supply chains, such as demand forecasting,
planning, sourcing, production, and distribution, to save millions of dollars.
It would be only natural to assume that these companies had the resources
necessary to commit themselves to such an endeavor: ERP platforms, or other
transactional systems that had amassed large amounts of historical data
(volume), and other sources of unstructured data (web clicks, GPS or mobile
data, etc); analytic tools, knowledge (both domain, and of methodologies, and
techniques), and the people to apply that knowledge to those tools; and money –
large amounts of money.
A
white paper by Inmon Consulting [i] suggests
that for a large data warehouse (one of two major platform architectures that
can be used to implement big data analytics; the other being Hadoop), say
several terabytes (1 terabyte = 1024 gigabytes) being managed by solutions
provided by IBM or Teradata (vendors), the cost of the infrastructure would be
$500,000 to $1,000,000 per terabyte. That is serious money.
On
the other hand, according to a Winter Corporation report [ii] on
the cost of big data, Hadoop, which is a lower cost, open source alternative to
a data warehouse, has a commonly quoted acquisition cost of $1,000 per terabyte.
That is still serious money.
These
are just the costs of acquisition. Factor in maintenance, upgrade, and other
recurring costs; throw in the cost of investing in human capital that can
actually maintain these monster data stores and do the analytics on them; and
you have huge overheads that inflate the initial acquisition figures. This is
daunting for any business – big or small.
However,
this money is something that big businesses can afford to invest. What about
small businesses? Are they going to be left out of the wave of big data
analytics sweeping across the business landscape and find themselves at an
inherent comparative disadvantage?
There
are many resources out there for small businesses that do not have the kinds of
fat purses their larger competitors have, to take advantage of analytic
capabilities for their data. Google Analytics is just one such tool that helps
business owners see who comes to their site, what pages, and what
products they look at, and what the overall traffic pattern is like. Use Google Analytics
with Google AdWords, add Facebook Insights into the mix, and you realize that
there are tools out there that democratize the availability of big data
analytics, and bring small businesses into the game.
Need
something more advanced or tailored than that? How Small Businesses Can Mine Big Data, an excellent article by
Christopher Null [iii] provides some pertinent examples of how small businesses can shop around for, and choose from amongst
different, lower cost big data service providers. Another article, How Can Your Small Business Use Big Data?, by Tim Parker [iv]
provides examples of services such as SumAll. SumAll acts like an aggregator
and takes data from different online platforms, such as Facebook, Twitter,
Tumblr, as well as Google Analytics, AdWords and AdSense and organizes it in “an
easy-to-read snaphshot” – and its free. There are other suggestions for using
your CRM data, or looking into government data. Square, [v]
which is a cheap payment processing option for small businesses, provides an
analytics dashboard for free. It can help small store owners answer questions
like what is the busiest time of day, which products are doing well, when
should they have the most staff, and so on.
Then
there are the many creative ways in which small businesses are trying to meet
resource requirements to be able to do big data analytics. Taken from a Harvard
Business Review blog article, [vi]
the following excerpt highlights that:
“True that in the past,
companies seeking to tap into big data needed to purchase expensive hardware
and software, hire consultants, and invest huge amounts of time in analytics.
But trends such as cloud computing, open-source software, and software as a service
have changed all that. New, inexpensive ways to learn from data are emerging
all the time.
Take Kaggle,
for instance. Founded in 2010 by Anthony Goldbloom and Jeremy Howard, the
company seeks to make data science a sport, and an affordable one at that.
Kaggle is equal parts funding platform (like Kickstarter and Indiegogo),
crowdsourcing company, social network, wiki, and job board (like Monster or
Dice). Best of all, it’s incredibly useful for small and midsized businesses
lacking tech- and data-savvy employees.
Anyone can post a
data project by selecting an industry, type (public or private), participatory
level (team or individual), reward amount, and timetable. Kaggle lets you
easily put data scientists to work for you, and renting is much less expensive
than buying them.
Online automobile
dealer Carvana,
a start-up with about 50 employees, used Kaggle to offer prizes
ranging from $1,000 to $5,000 to data modelers who could come up with ways for
Carvana to figure out the likelihood that particular cars found at auctions
would turn out to be lemons. For
the cost of the prize money (a total of $10,000), Carvana got “a hundred smart
people modeling our data,” says cofounder Ryan Keeton, and a model that the
company was able to host easily and inexpensively.”
Summing
it all up – there are many opportunities out there for the small business to
leverage resources and look into the data that they have. Looking at the picture below (a hardware vendor, somewhere in
Pakistan) – can all of them really do that?
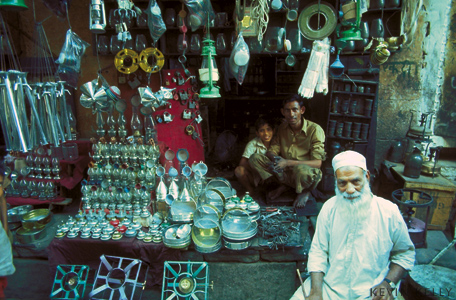
Image Source: http://asiagrace.com/pakistan/hardware-store.php
[ii]
https://www.google.com/url?sa=t&rct=j&q=&esrc=s&source=web&cd=4&cad=rja&ved=0CEAQFjAD&url=http%3A%2F%2Fwww.teradata.com%2Fwhite-papers%2FWinterCorp-Special-Report-Big-Data%2F%3Fpid%3D6478&ei=qGgOU_PULPS30AHQuYGoBg&usg=AFQjCNEZXhxz_s2dzS8BfTzNjmXjZt-6MQ&sig2=lyfqRjrUyeYSOO8HV9OUTg&bvm=bv.61965928,d.dmQ